In today’s rapidly evolving technological landscape, artificial intelligence (AI) is transforming industries and redefining business models. This revolution spans across various sectors. Whether it’s finance, healthcare, transportation, or advertising, AI is transforming traditional business models and demanding a new framework — the AI Business Model. Companies are leveraging AI to drive innovation, enhance efficiency, and create new revenue streams. This article provides an introduction to AI business models, highlighting their key components, types, and the impact they have on modern enterprises.
What are AI Business Models?
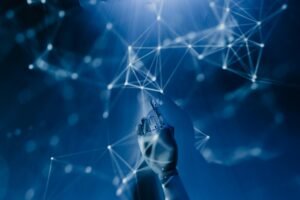
Understanding and leveraging the power of AI goes beyond just technical skills; it requires a thorough comprehension of how AI integrates with business strategies, workforce dynamics, and ethical considerations. This is the core of an AI Business Model — a framework that sees AI not just as a tool, but as a crucial part of business operations and strategies. These models focus on utilizing AI to solve problems, optimize processes, and deliver innovative products and services. At the core of an AI business model lies the use of data, algorithms, and machine learning to drive decision-making and business growth.
Key Components of AI Business Models
Data
The foundation of any AI model is data. Companies collect and analyze vast amounts of data from various sources such as customer interactions, social media, sensors, and transaction records, to train AI algorithms. High-quality, diverse data sets are crucial for building effective AI systems that learn patterns, make decisions, and improve over time.
Example: An e-commerce company collects data on customer behavior, purchase history, and feedback to personalize recommendations and improve customer satisfaction.
Algorithms
Algorithms are the core computational processes that transform data into actionable insights. They enable AI systems to analyze information, identify patterns, predict outcomes, and automate tasks. There are various types of AI algorithms, each suited for different purposes.
Key Types:
- Machine Learning (ML): Algorithms that improve automatically through experience.
- Deep Learning (DL): A subset of ML that uses neural networks with many layers to analyze complex data.
- Natural Language Processing (NLP): Algorithms that understand and generate human language.
Example: A finance company uses ML algorithms to detect fraudulent transactions by analyzing spending patterns and flagging anomalies.
Technology Infrastructure
A robust technology infrastructure is crucial to support AI applications. This includes hardware and software components that enable data storage, processing, and analysis. Cloud computing is often used to provide scalable and flexible resources for AI tasks.
Human Talent
Skilled professionals are essential to design, develop, and maintain AI systems. These experts include data scientists, AI engineers, and machine learning specialists who understand the intricacies of AI technologies and can innovate and troubleshoot effectively.
Ethics and Governance
Implementing AI ethically is critical to avoid biases, ensure privacy, and maintain public trust. Governance frameworks help manage the ethical use of AI, set guidelines for its development, and mitigate risks associated with its deployment.
Key Considerations:
- Bias Mitigation: Ensuring AI models are fair and unbiased.
- Data Privacy: Protecting sensitive information from misuse.
- Transparency: Making AI processes and decisions understandable to users
Types of AI Business Models
There are several types of AI business models that organizations can adopt, each with distinct characteristics and applications:
1. Product-Based AI Models:
Companies develop AI-powered products or services that are sold directly to consumers or businesses. Examples include AI-driven software applications, smart devices, and automated customer service tools.
Example: Smart home devices like Amazon Echo use AI to provide voice-activated assistance, transforming how users interact with technology at home.
2. Service-Based AI Models:
Businesses offer AI as a service (AIaaS), providing AI capabilities through cloud-based platforms. This model allows other companies to integrate AI into their operations without extensive in-house development.
Example: IBM Watson offers AI services through the cloud, enabling businesses to incorporate advanced AI capabilities into their operations with ease.
3. Platform-Based AI Models:
AI platforms enable the development and deployment of AI applications. These platforms provide tools, frameworks, and APIs that facilitate the creation of custom AI solutions.
Example: Google’s TensorFlow is an open-source platform that supports the development of machine learning models, widely used by researchers and developers.
4. Data Monetization Models:
Organizations leverage their data assets to generate revenue. This involves selling data, offering data analytics services, or creating AI models that provide insights from proprietary data.
Example: Social media platforms like Facebook analyze user data to offer targeted advertising services, generating significant ad revenue.
5. Integrated AI Models:
AI is integrated into existing business processes to optimize operations and improve efficiency. This model is common in industries such as manufacturing, logistics, and healthcare.
Example: Tesla integrates AI in its manufacturing processes and autonomous driving systems, enhancing production efficiency and vehicle performance.
Impact of AI Business Models
The adoption of AI business models has a profound impact on various aspects of business operations:
1. Innovation and Competitive Advantage:
AI enables companies to innovate by developing new products, services, and business processes. Organizations that effectively leverage AI gain a competitive edge in their markets.
Question: Have you ever wondered how Netflix predicts your viewing preferences so accurately? By leveraging AI algorithms, they provide personalized recommendations, keeping you engaged and satisfied.
2. Operational Efficiency:
AI automates repetitive tasks, enhances decision-making, and optimizes resource allocation. This leads to increased productivity and cost savings.
Example: In banking, AI-powered chatbots handle routine customer inquiries, allowing human employees to focus on more complex issues.
3. Customer Experience:
AI-driven insights help businesses understand customer preferences and behavior, enabling personalized marketing and improved customer service.
Question: Imagine receiving a special offer just when you need it. That’s AI enhancing your shopping experience by predicting your needs.
4. Revenue Generation:
AI opens up new revenue streams through the development of AI products, services, and data monetization strategies.
Example: Healthcare companies use AI to develop predictive analytics tools that help in early disease detection, creating new revenue opportunities.
5. Risk Management:
AI models can predict and mitigate risks, enhancing the organization’s ability to manage uncertainties and respond to market changes.
Example: Financial institutions use AI to detect fraudulent transactions in real-time, protecting both the business and its customers.
Conclusion
Understanding AI business models is essential for organizations aiming to harness the power of AI for growth and innovation. By integrating AI into their operations, companies can drive efficiency, enhance customer experience, and create new revenue opportunities. As AI continues to evolve, businesses that adopt and adapt AI business models will be well-positioned to thrive in the digital age.
Share your thoughts on AI business models in the comments below